Invited Speaker
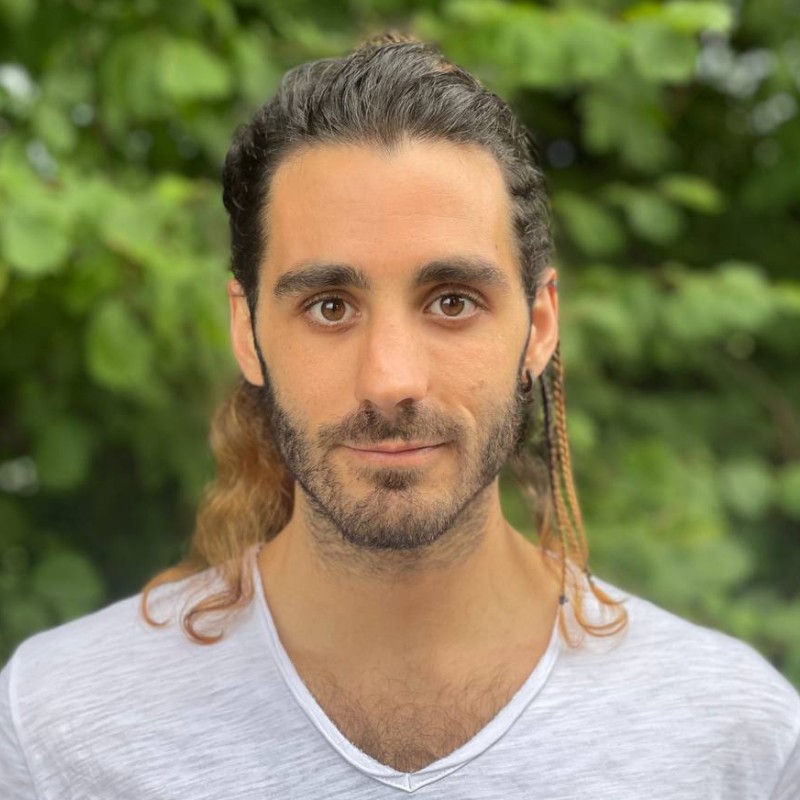
Mr. Carlos Hernández-Pérez
Universitat Politècnica de Catalunya, SpainSpeech Title: Interpreting Machine Learning models for Survival Analysis: A study of Cutaneous Melanoma using the SEER Database
Abstract: In this oral presentation, we delve into the application of three distinct machine learning algorithms for Survival Analysis: Random Survival Forest, DeepSurv, and DeepHit. It focuses on modeling cutaneous malignant melanoma using the SEER database. To enhance interpretability, we employ the SurvLIMEpy library, a Python package specifically designed for explainability in survival machine learning models. By leveraging these algorithms and explainability methods, we surpass the performance of the traditional Cox Proportional Hazards Model. Our findings shed light on the significance of explainability techniques in deciphering black-box models and offer valuable insights into the crucial features influencing melanoma prognosis.
Biography: Carlos Hernández Pérez is a Telecommunications Engineer and a pioneering Ph.D. candidate at Universitat Politècnica de Catalunya, specializing in Artificial Intelligence applied to Biomedicine. His innovative research is characterized by the development of cutting-edge image-processing techniques for crucial medical applications. As a co-founder of the IoT-based Fireback project for forest-fire prevention and an Independent AI Consultant, Carlos has successfully bridged the gap between academia and industry. He is the creator of the SurvLIMEpy library, a significant contribution to interpretable machine learning for survival analysis.