Invited Speaker
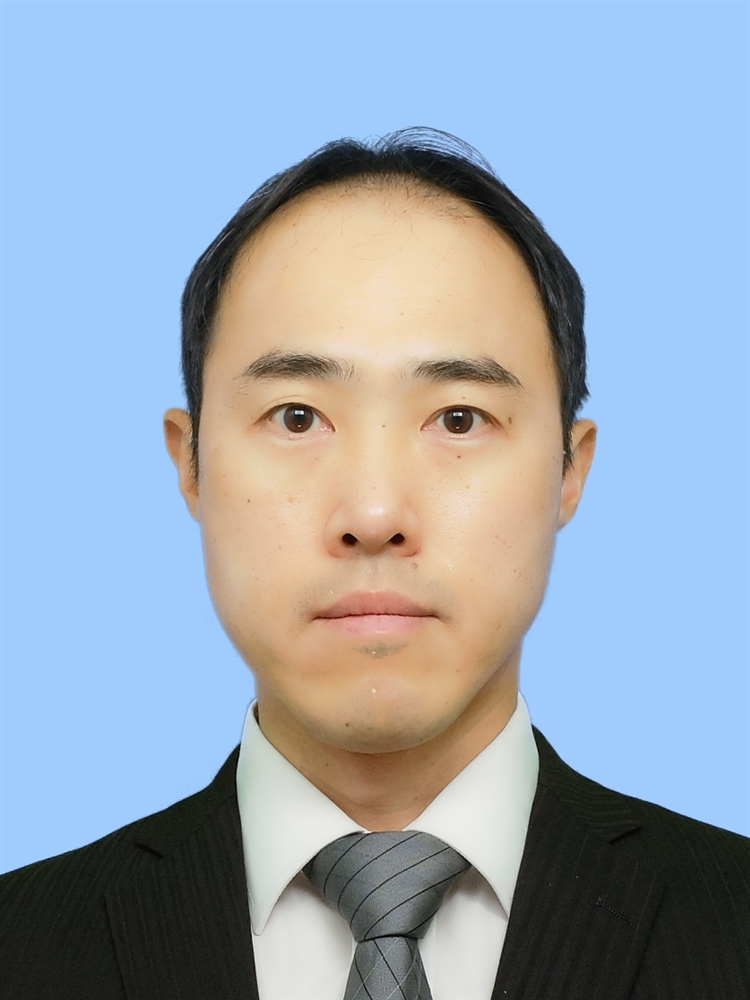
Prof. Hirotada Honda
Faculty of Information and Networking for Innovation and Design, Toyo University, JapanSpeech Title: On the learnability of differential equation-based neural networks
Abstract: Recently, differential equation-based neural networks have attracted considerable attention. An important contribution is ODENet, which proposes a learning framework comprising a system of ordinary differential equations working as the middle layer of a neural network. Furthermore, the application of partial differential equation has also been observed. In this talk, we shall discuss the agnostic PAC learnability of a differential equation-based neural network in a supervised learning framework. Motivated by recent developments of the differential equation-based neural networks and related works, we will consider its statistical learning theory perspective; in particular, the effectiveness of the empirical risk minimization approach. We shall also introduce recent Bayesian approaches in relation to the learnability perspective, and our Bayesian optimization approach as well.
Biography: Hirotada Honda received the Ph.D. degree in mathematics from Keio University in Japan in 2011. From 2002 to 2018, he worked for Nippon Telegraph and Telephone Corporation (NTT). Since 2018, he has been working for Toyo university in Japan, and currently, professor in Faculty of Information and Networking for Innovation and Design (INIAD) of Toyo University. His research interests include statistical learning theory, mathematical optimization, application of functional analysis and differential equations to machine learning theory.